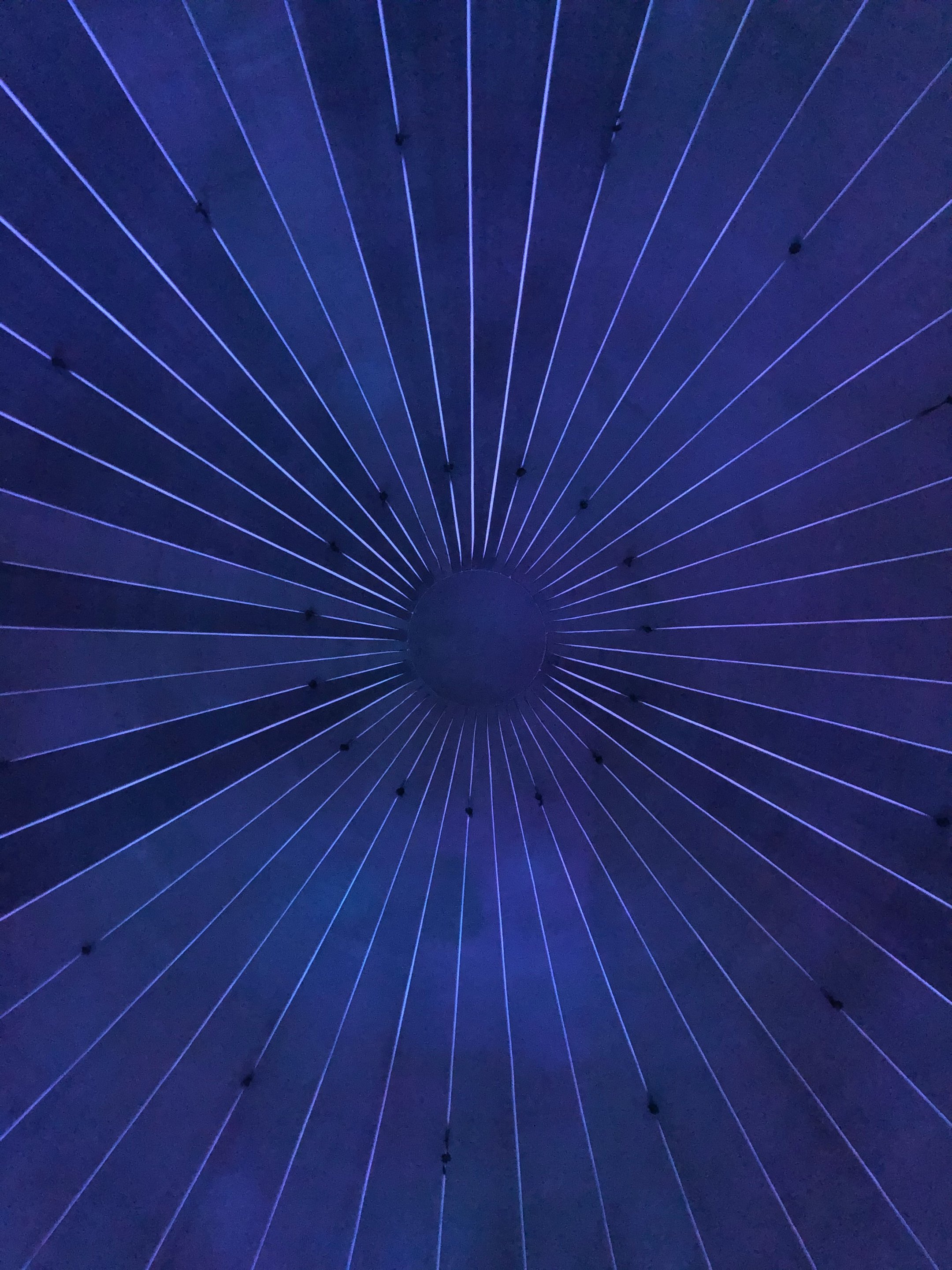
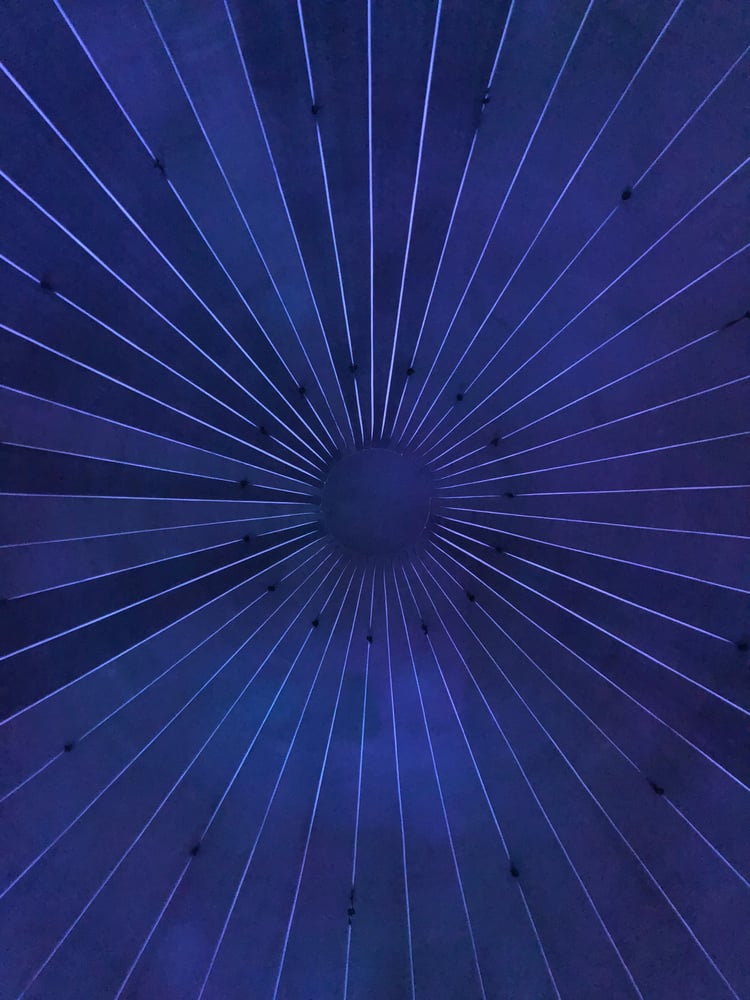
Reading the news or scrolling through social media, you might think that artificial intelligence (AI) was just invented. In reality, products with embedded artificial intelligence have been around for decades.
So why the sudden interest? Generative AI. Let's look at the two most popular AI tools.
Generative AI for text-based content
The release of ChatGPT seems to have unleashed a torrent of interest in AI. People are experimenting with the range of things we can do, from conducting research to streamlining business functions, from writing code to writing business plans.
ChatGPT is the most recognisable name in Generative AI, though every major technology company has released their own Large Language Model (LLM). The premise behind an LLM involves training algorithms to generate new data, images, and/or text that mimics human creativity and intelligence. Generative AI uses machine learning models that learn from large datasets to generate new data points based on patterns and relationships in the data.
The important word in the previous paragraph is "mimic". Because ChatGPT bases its results on its training data sets, it is essentially recombining information from its training data and regenerating it for a new context. In other words, the results can tell you what's already been said. Or, as sceptic writer Neil Gaiman says, "ChatGPT doesn't give you information. It gives you information-shaped sentences." (Neil Gaiman on Twitter).
Generative AI and visual content
The interest in ChatGPT is not to diminish the interest that image-generation AI such as DALL-E has generated. (Google results show some 82 million articles on the topic, though the article count about ChatGPT stands at 311 million at the end of April.)
Generative AI is a subset of deep learning. It's called generative because it builds systems that can generate new information by learning from existing information. These systems are trained on massive amounts of information, and work by predicting the next logical pixel to produce a new digital asset such as a new image.
An AI text-to-image generator uses a machine learning technique - machine learning is a subset of AI - called artificial neural networks. It mimics the human brain in that you input words, and the AI "translates" the words, through a specific type of processing, to generate an image. It does that in a matter of seconds. However, the reliability of the results correlates the amount of training that the artificial neural network has had. It's similar to teaching small children the terms for objects in their world. This is the same process that AI generators go through during training, but at a much faster speed with a lot more data. And if you have ever seen the chihuahua or muffin meme, you'll know that object classification for machines isn't as easy as it may seem.
How much of AI is just hype?
Every new technology comes with a promise and elicits a dream. But how much of what's been written about generative AI is reality and how much is hype?
Long before generative AI exploded, AI has been quietly implemented in various sectors. A fascinating example is a set of AI-embedded tools that comprise a smart platform for the grocery distributor, Ocado. AI calculates a vast, complex range of data to forecast, schedule, and generally provide superior service to customers. Another aspect of the platform also uses AI to help its robots pick and pack groceries, with allowances for relative weight (heavy items in the bottom of the bag), relative bulk, and overall size (for carrying by humans).
Another example is a company that started in 2002 in a German research centre for artificial intelligence. It started as a content quality checker, grew to content optimisation - checking spelling, grammar, voice and tone, standardisation, readability, and even spotted duplicate content intent when the content was written very differently - and now handles content governance. The sophistication needed to carry out the jobs of multiple editors at lightning speed and near-perfect consistency is staggering.
What Generative AI can and can't do
There's so much to say that it will take more than a single article to say it. This article is the first in a series where we will explore the impact that generative AI will have in the areas of visual content and text-based content, and focus on the areas of marketing, life sciences, and product content.
What we will look at are existing uses and the potential that generative AI can bring to businesses, recent changes in content operations as a result of generative AI, limitations, and ethical and copyright considerations. We hope you'll follow us on this fast-paced journey, where it seems that every week brings new twists and turns to its course.
Rahel Bailie, Executive Consultant EMEA
Over the coming weeks, we will be publishing 5 further blog posts on the topic of AI: AI use with Visual Content, AI use with Text-Based Content, AI use in Marketing, AI use in Life Sciences, and AI use for Product Content.