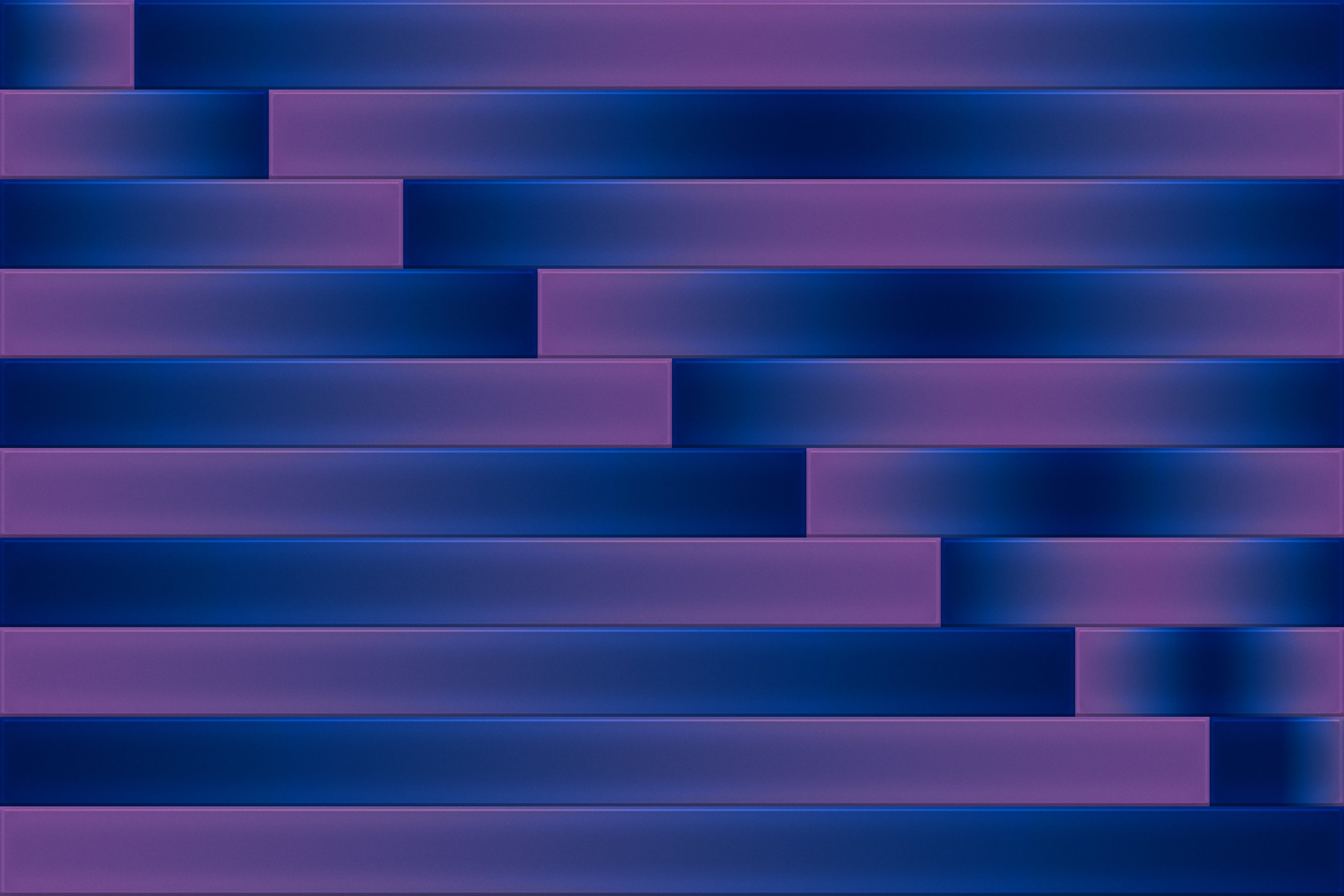
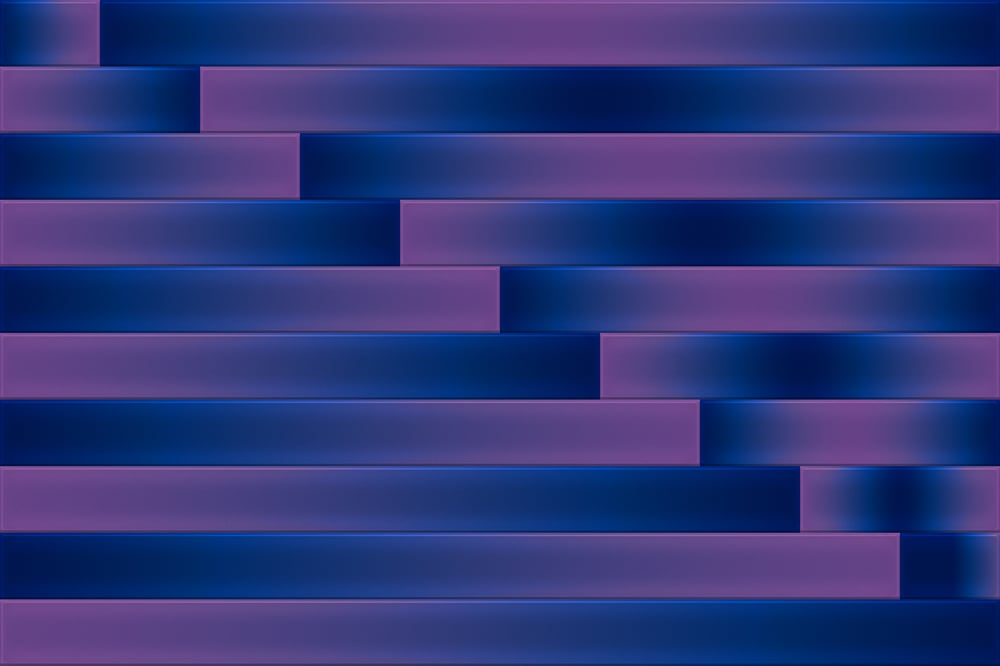
This is the third instalment in our series about Generative AI. You can find our previous posts here: Artificial Intelligence, Navigating the shifting sands and Generating visual content using AI.
When it comes to discussing AI text generators - in other words, generative AI for text-based content - discussing all the facets and trends could fill an entire book. For this article, let's focus on generative AI in three areas: marketing, life sciences, and product content. It's likely we will only be able to skim the surface of the vast potential that AI can bring in terms of both operations and innovation, but that's a good start.
Technology is moving fast
ChatGPT has only been around for a few months, and it already seems old news. Open AI moved on to GPT-4 and a number of new AI text generators are competing for space in the market. Trends are moving so quickly that the open source Large Language Models (LLM) must already compete with proprietary LLMs. Alberto Romero of The Algorithmnic Bridge lays out how incumbents are incorporating AI into existing digital products - for example Google's embedding of their generative AI into Search and Workspace, and Microsoft's beefing up Bing, Edge, and Office applications. There is also strong speculation that smaller language models will provide smaller, specialised, customised models specific to a domain as an alternative to LLMs.
How is generative AI currently being used
There is a significant difference between what generative AI seems to do, what it can do, and what it should be used to do. In the past two months, we have examples of each of these.
What AI seems to do
In a court case that has gone viral, a US-based lawyer presented legal research that a colleague had assembled using generative AI. However, ChatGPT invented several false citations, and when asked, ChatGPT asserted that the cases were real and could be found on the LexisNexis database. What started out as a time-saving endeavour has turned into a disciplinary hearing for the humans involved. It is worth noting that there is no corresponding sanction for ChatGPT, despite the model technically being the entity that cheated and lied about it. The term to describe this phenomenon is called "hallucination" and experts are already publishing articles on how to prevent LLM hallucination by creating more contexts with prompts (called prompt engineering).
Similarly, there has been much speculation about the ability of generative AI to pass a US medical exam. However, when given less formulaic sets of conditions by an actual doctor, the diagnoses were far less reliable. It seems that with more training, the diagnoses get better, according to one medical lecturer, which is encouraging. Evidently GPT-4 has a better track record at diagnosing rare diseases, which makes it a valuable assistant to a doctor.
But given the tendency of ChatGPT and GPT-4 to invent facts, it would be risky to encourage its use by the public, the way that some AI-driven symptom-checking apps are available for use.
What generative AI can really do
AI can generate documents that are grammatically correct. As Neil Gaiman said on Twitter, "ChatGPT doesn't give you information. It gives you information-shaped sentences." One commentator replied that there’s tremendous value in “information shapes” as the alternative is what we currently do: listen to words and THEN manually shape it ourselves; having information PRESHAPED by AI is more energy efficient with some LOSSINESS as a trade off.
This distinction is important because the documents generated tend to come across as generic and rather uninspired. (As an aside, an agency provided an AI-generated white paper on a specialty topic in record time, clearly enamoured with the power of the technology. The client, however, was not impressed and told them that they could have done that themselves, and if they wanted to get paid, to come back with some evidence of "real" work being done.)
What generative AI can do is act as a research intern. In other words, AI can generate a lot of ideas to use as fodder. It's important to then sift through the content generated to determine what is valuable and what to discard.
Another thing that generative AI is getting quite sophisticated at is prompt engineering. Current thinking (as of summer 2023, at least) is that getting good results from an LLM is done through a conversation that provides increasing amounts of context. In effect, it's a little like talking to a child. Here's a simple example:
Adult: Tell me how you made the mess.
Child: I was cleaning my room.
Adult: Tell me how you made the mess in the kitchen.
Child: I was making cookies.
Adult: Tell me what kind of cookies you were making when you made the mess.
Child: I made oatmeal cookies, peanut butter cookies, and mud cookies.
Adult: Don't tell me about cookies that use things from outside the kitchen.
Child: Only the oatmeal cookies don't have any mud in them.
In this hypothetical example, the more context is provided, the better the results.
In an advanced scenario, prompt engineers can create prompt templates to allow for prompt storage, re-use, sharing, programming, and chaining. This automates the prompts for queries that might be repetitive, such as gathering the same sort of marketing analytics information for multiple brands. Extending this example, AI can be used to gather more sophisticated analytics by experimenting with the context through the smart use of prompts. The capabilities for search makes AI search queries a powerful tool in any marketer's toolkit.
When generative AI could and should be used
Generative AI has applications in many fields, from business applications, scientific research, and artistic creation, as long as there is adequate supervision by a qualified professional. For example, in business applications, generative AI can be used to generate marketing content. When using an LLM that is hooked into a company's content corpus, generating content such as the definitive feature list of a product becomes very time-efficient. Writing emails or other customer-facing communications that conform to a particular tone or voice are also easily done.
AI is already helping with customer service; chatbots using generative AI autonomously carry out tasks such as taking restaurant reservations - and the chatbot never forgets to ask about allergies or special occasions. For the bulk of customer service queries, the AI can route requests to the proper Call to Action, such as a password reset, surfacing the right knowledge base article, or routing the caller to the right person to handle their query.
Writing product descriptions is a good example of how to put generative AI to use. One online retail platform already has a tool called Shopify Magic that promises to create compelling product descriptions in multiple languages for online products. Natural Language Generation analyses product data and generates a description, complete with features and benefits, that can match a brand's voice, and even change tone appropriately within that voice.
Doctors are using generative AI to lighten their workload, specifically when writing up patient notes. In fact, one study showed that patients preferred the AI-mediated responses to those of doctors, citing more empathy.
What all of these examples point out is that generative AI can be a powerful technology bringing significant value. However, it does need ongoing training, supervision, and care.
Limitations and cautions of AI use
The saying "with great power comes great responsibility", and that sentiment is so true when it comes to using generative AI. In a TED talk about AI and ethics, Zeynep Tufekci gives multiple examples of algorithmic bias that has led to gender bias in hiring, racial bias in predicting offender re-offense, and financial bias on Wall Street. These are examples of ethical considerations, such as the potential misuse of generated content or bias in the generated data. Data privacy concerns may also arise when generating content from user data. Your doctor may save time writing patient notes, but you certainly don't want them using the public version of ChatGPT to do so, where your medical history becomes a training input.
Technical limitations, such as the amount of computational power required to train models, may also be a constraint. The carbon footprint of generative AI is extremely heavy - it uses more energy than any other type of computing - and if the predictions about how many data centres need to be built and how fast, is accurate, the potential for generative AI to contribute to global climate change is alarming.
Making AI a trusted technology
When AI generates new visuals, the biggest obstacle is copyright. When AI generates new text, there is so much more at risk. Breaching copyright is much less of a risk than when an LLM hallucinates: misrepresentation, misdiagnosis, misinformation, algorithmic bias, and leaking of company secrets are all possible outcomes.
We can look to Microsoft, who have offered a model to foster responsible and trusted AI: ethical and explainable. The six key principles for responsible AI are accountability, inclusiveness, reliability and safety, fairness, transparency, and privacy and security. Incorporating these into a strong governance model will promote the use of generative AI while preventing the types of events that trigger embarrassing situations and inclusion in the AI Hall of Shame.
The idea is to mitigate potential risks associated with using generative AI - for example, the generation of inappropriate or harmful content, the perpetuation of bias in generated data, and the difficulty of controlling the generated content. Ways to address these risks may include setting up safeguards and monitoring systems to detect and remove inappropriate content, and implementing measures to reduce bias in the generated data. An AI ethicist may soon become as common a job as a prompt engineer. A company should be having regular conversations, not just strategising about the threats and benefits, but also about the ethics of the development and use of AI.
Rahel Bailie, Executive Consultant EMEA
Over the coming weeks, we will be publishing three further blog posts on the topic of AI: AI use in Marketing, AI use in Life Sciences, and AI use for Product Content.