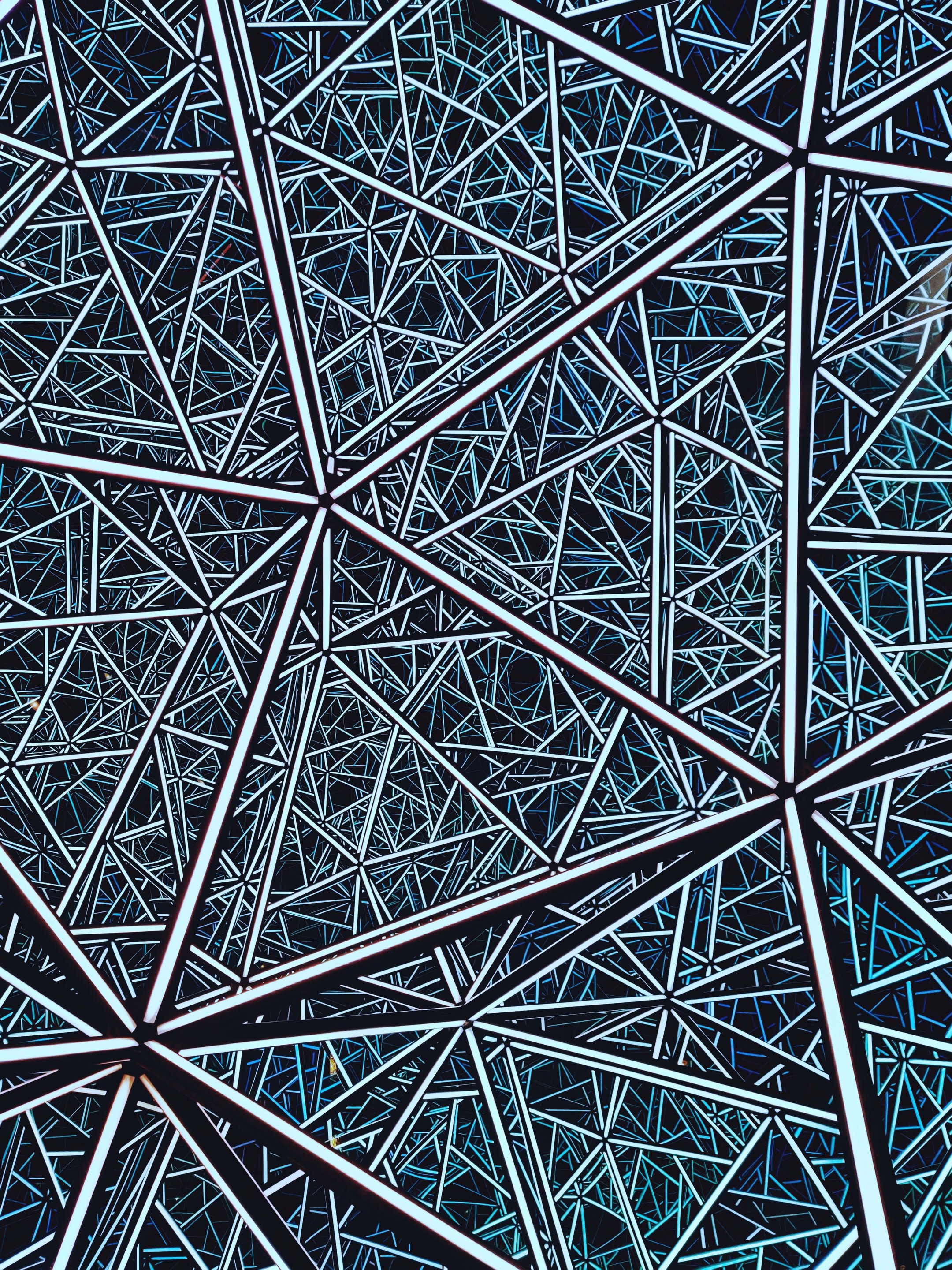
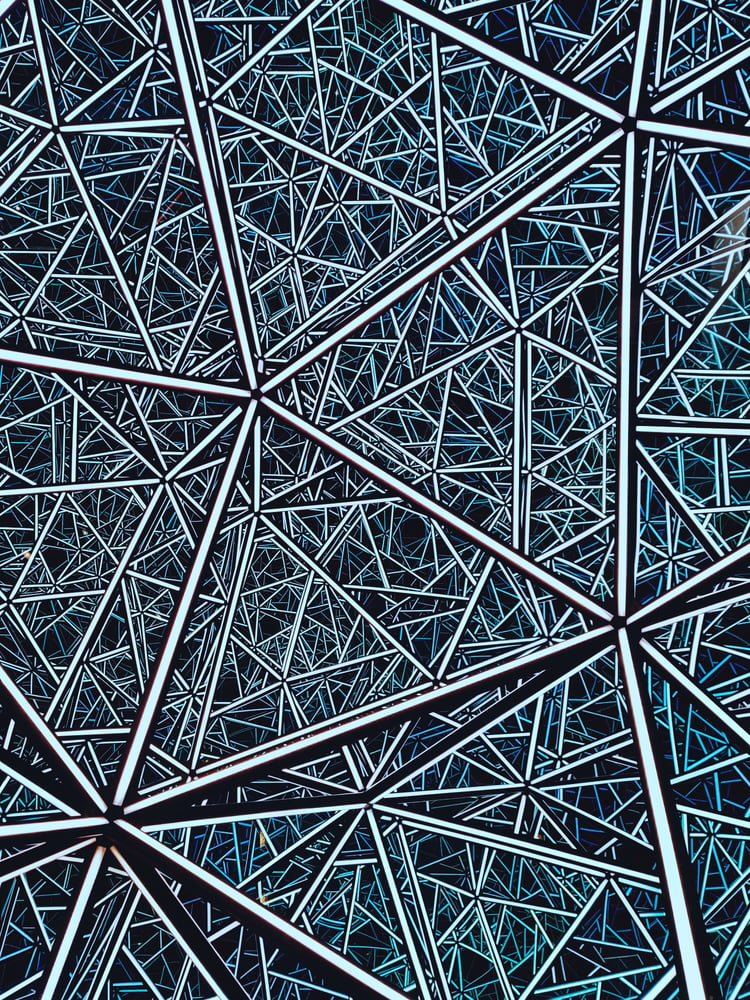
This is the fourth instalment in our series about Generative AI. You can find our previous posts here: Artificial Intelligence, Navigating the shifting sands, Generating visual content using AI and Applying Generative AI to text-based content .
Marketing departments are, understandably, looking for ways to use Artificial Intelligence (AI) and now generative AI (GenAI) to improve operations, gain competitive advantage, and ultimately drive business growth.
The current hype would have us believe that marketers can take care of all of their content creation needs by having ChatGPT generate never-ending copy. Sure, there are "junk websites" that are filled with subpar content that exist purely to generate income from advertising, but reputable brands are most likely to be the victims rather than the perpetrators – when a brand counts on a service to match personalised ads to content, they often don’t know where their ads appear. The truth is way more nuanced than that. Here are a few ways that marketers have been using AI to help marketing efforts and generative AI to boost productivity.
Using traditional AI in marketing
It may sound incongruous to use the term "traditional" in reference to AI, but the reality is that there have been AI tools developed over a decade ago that have been used by marketers. Many of these tools are still in use, with new ones coming onto the marketplace almost daily. Companies such as HubSpot, Jasper, Grammarly, Synthesia, and Salesforce Einstein are all examples of tools within a marketing tech stack that are enhanced with embedded AI. Now that Large Language Models (LLMs) such as ChatGPT have gained prominence, technology vendors have been quick to find ways to incorporate those capabilities into their products.
AI for market segmentation
Market segmentation is the grouping of prospective or existing customers with common market needs and behaviours. The parameters for differentiating groups are:
- Geographic. This means segmenting by users' locations, whether that is by legally-defined areas such as a country, state, or province, a density factor such as country vs city, or natural factors such as customers who live next to bodies of water.
- Behavioural. This type of segmentation relies on past behaviour to predict future behaviour, particularly relating to product purchases: what do they buy and how do they decide what to buy and when.
- Demographics. Segmentation here is by socio-economic factors such as age, gender, income levels, family status, and other aspects that can affect responses to marketing.
- Psychological. These are probably the most amorphous factor to categorise. The categories might not be obvious. They might be by beliefs, hobbies, likes and dislikes, or even personality traits.
AI can help identify market segments that aren't intuitive. At first glance, a group may seem to have quite disparate interests but are bound together by some less obvious thread. It takes AI far less time to identify such affinities and can make suggestions that we wouldn’t think of.
AI and business intelligence
Business Intelligence (BI) refers to sifting through large data lakes - that is, masses of structured and unstructured data - to create insights. AI is a strong part of the power behind analysing the data in ways that reveal business insights - that is, intelligence. AI's “intelligence” is about computing power; the software can crunch through huge amounts of data with relative speed, which lays the groundwork for analysts to discover insights. In BI, "intelligence" is about business decision-making from the analysis of that data. This allows marketing departments to look at past performance to make data-driven decisions for future performance.
AI in personalisation
Personalisation is a coveted ability in marketing, as a way of connecting with customers and increasing engagement. Delivering personalised content such as product recommendations, dynamic pricing, and personalised messaging are just a few days that AI can help enable. Because AI has evolved to make it easier to understand things such as sentiment, context, conversational rules, and nuance, the potential to deliver conversation-worthy personal content is significant. This is of particular interest with chatbots that now have the ability to provide conversations that engage customers.
AI for image recognition and analysis
In a previous article, Generating visual content using AI, the benefits and cautions of generating images with AI were covered, so that topic won't get rehashed. Aside from actual image generation, AI can help marketers in other ways. GenAI can analyse visual assets - images, videos, infographics, and related images - to identify patterns, objects, and emotions. Doing so can auto-route specific images for targeted marketing campaigns and personalised image delivery to help users better connect with them. AI can also help with attribute tagging, which allows products to have extra metadata to help customers find the products they want. AI can also analyse customer interactions to better understand customer sentiment.
Multivariate testing
Generative AI makes it easier for marketers to generate multiple variants of content, and test that content at scale. Multivariate testing creates different combinations of content to see which combination performs best for particular audiences. This allows brands to optimise their campaigns and fine-tune their messages for optimal engagement.
Sentiment analysis
The business interest behind sentiment analysis is to understand customers' feelings about the brand and products or services. Rather than blunt-force techniques such as surveys and support tickets or complaints on social media, AI can analyse all sorts of communications from different sources and suggest course corrections that can avoid or allay problems.
Revenue operations
Revenue operations is a relatively new concept that can be best described as being related to marketing automation. It's an attempt to connect the efforts of marketing and sales, with AI involved in processing data across silos. This automates the data analysis in a way that helps with business decisions such as resource allocation, customer lifecycle optimisation, and sales forecasting to orchestrate cross-functional revenue.
Cautions about using AI for marketing purposes
As with any new technology, jumping in head first can have some unexpected consequences. Here are a few cautions to think about when embarking on this new adventure.
- Beware of the algorithms. AI algorithm can often be a black box. Only the AI engineers know how it's been built and sometimes they don't even know what biases the algorithm might have. This makes it challenging to understand how the model arrives at certain decisions or recommendations. At a time when customers can be quick to point out race, gender, and class biases, it's important to be accountable for outcomes that are fair and transparent.
- Scarcity of high-quality data. Making data-based marketing decisions is great … if you have good data. Despite all the hype about AI working with data lakes with structured, unstructured, and "uniquely structured" data, the results could possibly send your marketing efforts in an unanticipated direction. Keeping an eye on the results that come from data crunching is worth the effort.
- Ethical decisions. Copyright concerns were already discussed in an earlier article. As companies react negatively to the EU regulations being proposed, and multiple lawsuits against OpenAI brew in the US, it's important to consider the multiple ways that privacy, policy, regulatory, and other aspects related to ethics can boomerang back on our brands. Adopting a code of ethics is the first step; continuous training and monitoring of AI models is an ongoing concern.
Overall, the advantages of incorporating AI into the marketing technology stack look like they outweigh the negatives. We do need to remember, however, that it's only a tool, and the responsibility is on us as humans to ensure that the tools we choose are used wisely.
Rahel Bailie, Executive Consultant EMEA
Over the coming weeks, we will be publishing two further blog posts on the topic of AI: AI use in Life Sciences, and AI use for Product Content.